Credit Risk
Fragmented perspectives.
According to a study by the Federal Reserve Bank of New York, the cost of a fragmented credit risk profile can be as high as $300 billion per year in the United States. This includes the cost of higher interest rates, denied loans, and increased difficulty accessing credit.
McKinsey Global Institute found that digital transformation in credit risk management could save the financial services industry up to $100 billion per year by 2025.
Another study by the same organization found that digital solutions can improve the accuracy of credit risk assessments by up to 30%.
A study by the Federal Reserve Bank of New York found that the use of digital solutions for credit scoring can reduce the risk of default by up to 50%.
A study by the World Bank found that the use of digital solutions for credit risk assessment can help to increase financial inclusion by making it easier for people to access credit.
Data fragmentation: There are many different sources of data that can be used to assess a person's credit risk, but this data is often fragmented and siloed. This makes it difficult to get a complete and accurate picture of a person's financial situation.
Data quality: The quality of the data used to assess credit risk can vary greatly. Some data may be incomplete, inaccurate, or outdated. This can lead to inaccurate assessments of credit risk.
Data bias: The data used to assess credit risk can be biased. This is because the data is often collected from a limited pool of people, such as those who have a credit history. This can lead to unfair assessments of credit risk for people who are not represented in the data.
Privacy concerns: People are often reluctant to share their financial data with lenders and other organizations. This is because they are concerned about their privacy. This can make it difficult to get the data that is needed to assess credit risk accurately.
Our data apps.
Use data apps to integrate credit report data, public records data, employment data, income data, assets data property data and more, all rapidly in the most powerful data network. Connect to existing legacy systems and platforms.
Deploy useable prototype within 6 weeks.
-
Centralized data integration layer.
Integration from multiple sources: databases, data warehouses, cloud storage, API, and more.
Copyless integration.
-
Map & transform data from different formats and schemas.
Mapping and transformation of data between new and old data models seamlessly.
-
Common semantic model and leverage domain ontologies for universal data model.
Change schema without breaking application code.
Handle schema modification via data virtualization.
-
Data browser to search data network.
Expand and collapse data set relationships.
Ease of data exploration whilst visualizing data governance attributes.
-
Data lineage tracking, data masking, controls, security built in.
Create enterprise wide smart contracts to deploy in data fabric.
-
Store and structure data for rapid application development and use cases.
Encode real-world flows and interactions between physical assets.
360° Intelligence & Risk Card Scoring
-
Create a 360° View of Customers
Autonomous integration of information in a networked graph architecture ensures that all customer data is standardized, clean, and represented in a way that captures a very accurate and contextual view of the customer.
-
Improve Fraud Detection
Clean, standardized, contextual, and connected data provides accurate customer and agent profiles, thus enhancing predictions related to fraudulent activity in real-time (ie. agent gaming, faulty claims etc.).
-
Enhance Risk Modelling
Orbyfy's platform improves modelling of real world assets, and client risk profiles, resulting in improved predictions of black swan, and higher frequency medium risk events - all supported by blockchain, data fabric, and NFT technology. The net result: improved premium, expected claims, loan default models & predictions.
-
RT Monitoring of Assets & Clients
Orbyfy's low code data integration, pre-built governance, digital twins of assets, and IoT capabilities ensures real-time monitoring of insured physical machines, infrastructure, and customer information that drives 360 risk based visibility and decisioning across organizations.
-
Enhance Loss Ratio and Pricing Modelling
Clean and contextual customer, governance, and financial information ensures loss ratio, claims, and premium pricing predictions are more accurate and representative of the real world, thus improving reserve allocations, and hedging strategies and decision making.
-
Improve Scorecard Modelling
Enhanced customer profiles through real-time connected, and contextual data combined with bespoke or pre-built AI driven scorecard models produces a holistic risk based view of the customer that is ever evolving.
Features.
Bringing together data from different sources
Platform helps to bring together data from different sources, such as credit bureaus, banks, and social media. This can help to create a more complete and accurate picture of a person's financial situation.Improving data quality
Platform helps to improve the quality of the data used to assess credit risk. This can be done by using machine learning and other techniques to identify and correct errors in the data.Addressing data bias
Platform helps to address data bias by using techniques such as data sampling and machine learning. This can help to ensure that the data used to assess credit risk is representative of the population as a whole.Protecting privacy
Platform helps to protect privacy by using encryption and other security measures. This can help to ensure that the data is only used for the intended purpose and that it is not shared with unauthorized third parties.
Leverage our prototyping and incubation hub to quickly create dashboards and visualizations.
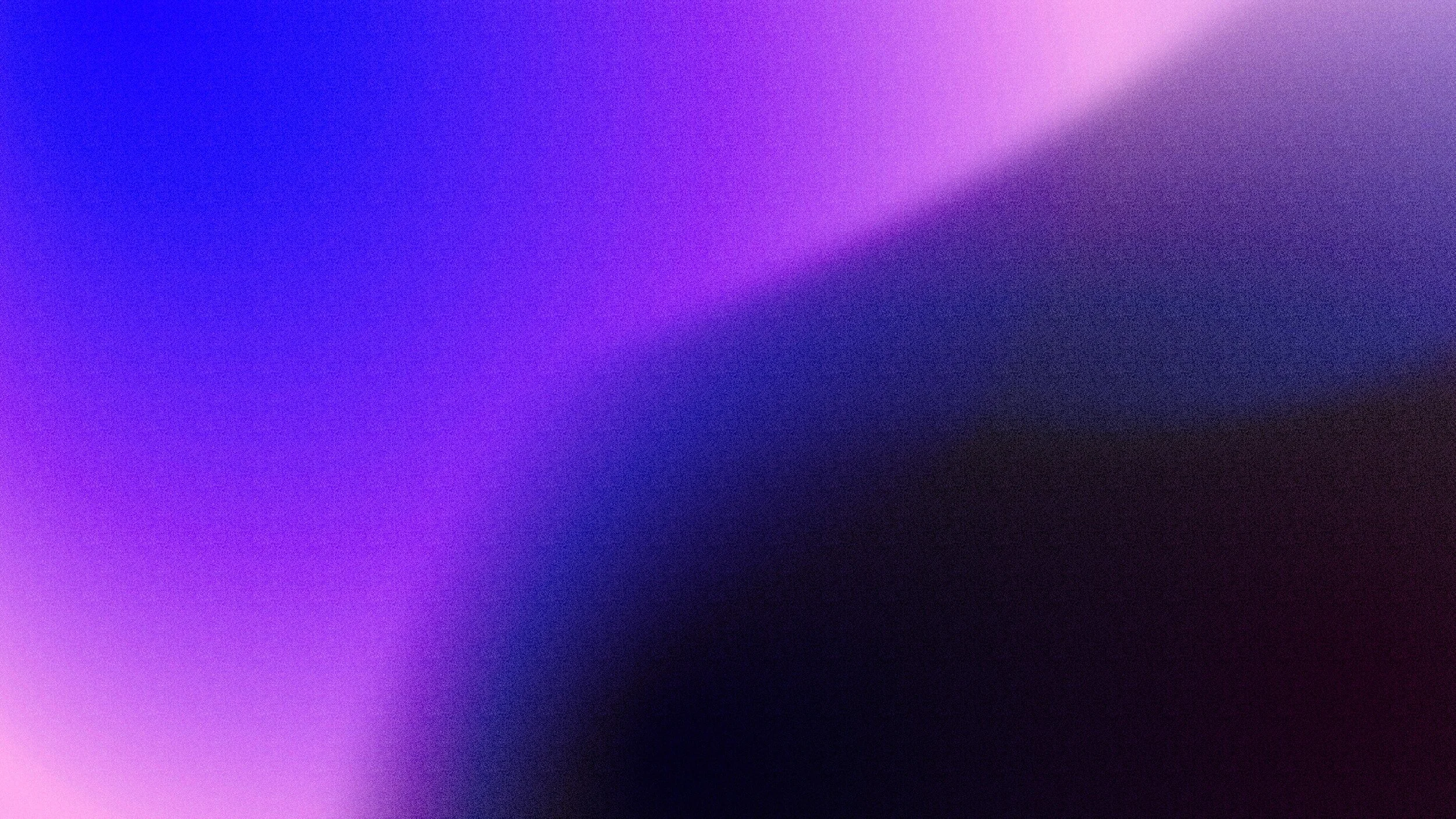
How do you begin?
Connect with out team and learn how we are building something different.