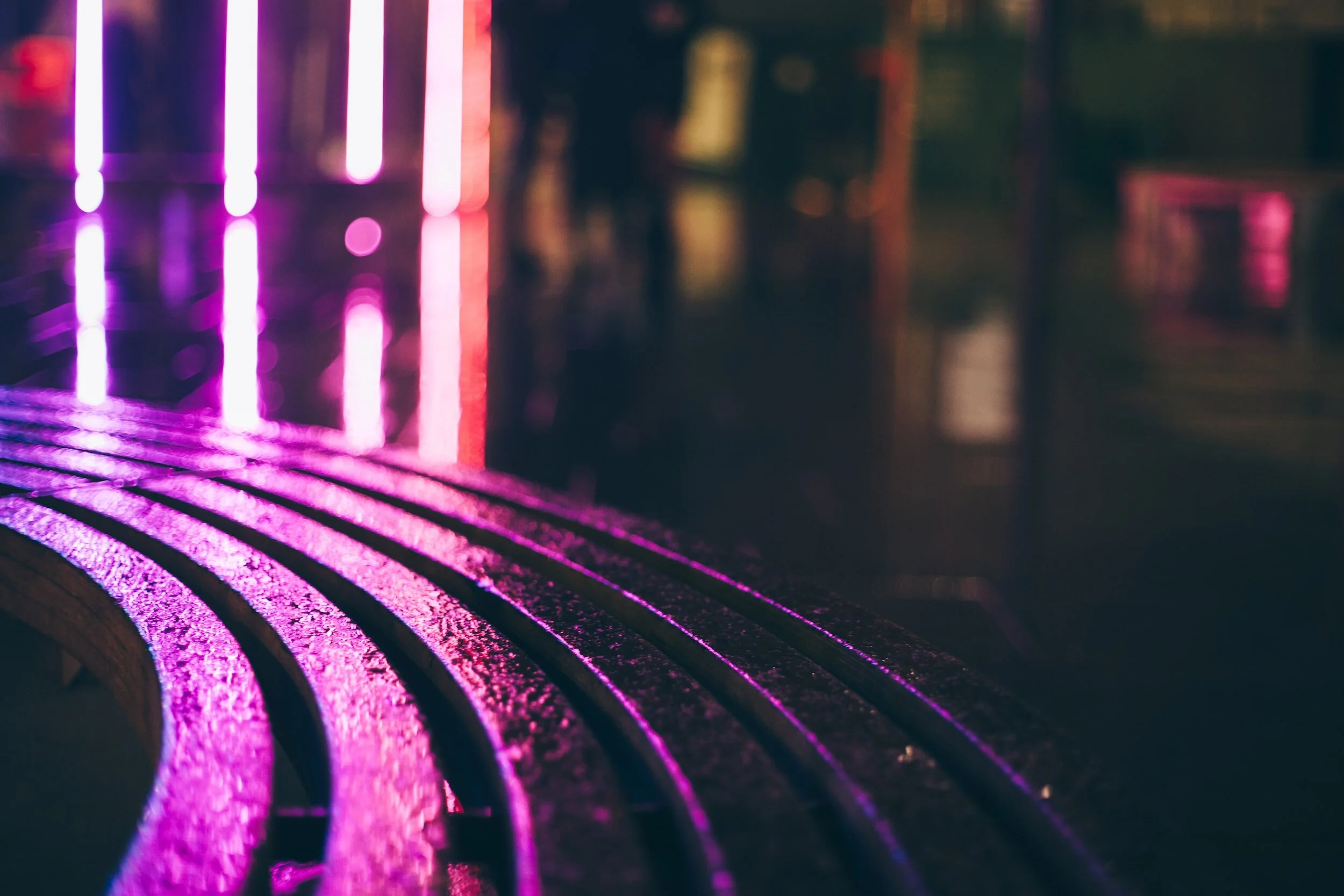
Insurtech innovation.
Digital twins that precisely simulate reality in a reactive real-time bi-directional, synchronous, high-fidelity, and in a closed-loop.
Current state of managing risk.
The process that insurance companies take in insuring industrial assets such as power generation equipment typically involves several steps to assess the risk and determine the appropriate insurance premium.
Firstly, insurance companies will conduct a detailed risk assessment of the industrial asset to identify any potential hazards or risks that may result in loss or damage. This may involve a site visit, inspection of the equipment and review of relevant documentation.
Next, they will analyze historical data and loss experience for similar types of assets to determine the probability of loss or damage occurring. This analysis will also consider external factors such as weather patterns, natural disasters, and economic conditions that may affect the risk level.
After determining the probability of loss, insurance companies will calculate the potential financial impact of a loss event, taking into account the cost of repair or replacement of the asset, as well as any potential business interruption losses.
Using this information, insurance companies will then set the insurance premium, which reflects the level of risk and financial impact of a loss event. The premium will be based on a combination of factors such as the location, age, condition, and type of the asset, as well as the risk profile of the insured party.
In order to manage the loss function and stay profitable, insurance companies will typically use a combination of risk management strategies. These may include implementing risk mitigation measures such as loss control inspections, requiring regular maintenance of equipment, and establishing safety protocols. Additionally, insurance companies may use reinsurance or other financial instruments to spread risk and reduce the financial impact of a loss event. They may also adjust their insurance premiums based on changes in the risk landscape or loss experience.
Overall, insurance companies use a combination of risk assessment, historical data, and financial analysis to determine the appropriate insurance premium for industrial assets such as power generation equipment. By managing their loss function through risk mitigation measures and reinsurance, they are able to remain profitable while providing coverage for their clients.
Insurance technology innovation.
The loss ratio has a profound impact on operating performance, as demonstrated in a McKinsey study. Over the past three decades, the top insurers have shown significant variability, with operating results being the primary driver of overall financial performance. The loss ratio plays a critical role in this, highlighting the need for advanced technologies such as big data, advanced analytics, and AI to drive underwriting excellence. These technologies can be utilized for optimal pricing adequacy, risk selection, and coverage design, all of which are key components of successful underwriting. Siemens technology is entirely focused on elevating this area by utilizing the data at hand to its fullest potential. By leveraging the latest technological advancements, we can enhance our ability to make informed decisions and achieve optimal outcomes, improving our overall performance in the long term.
The insurance industry is highly dependent on profitability, which is primarily determined by the loss ratio. To improve this ratio, insurance companies are looking to leverage Digital Twin and modeling and simulation technologies. This page will explore how these technologies can be utilized to improve profitability and what that entails, and how it is better than conventional approaches used in simple ‘data and analytics’.
Digital twins can be used to improve upon the loss ratio by providing insurers with better insights into their customers, risks, and claims. For example:
Digital twins can be used to create virtual models of physical assets, allowing insurers to better understand the risks associated with insuring those assets and make more accurate pricing decisions.
Digital twins can be used to analyze large amounts of data to identify patterns and trends that can help insurers predict and prevent losses before they occur.
Digital twins coupled with IoT such as sensors and cameras can be used to monitor assets in real-time, providing insurers with immediate alerts when a loss occurs and allowing them to respond quickly and effectively.
Digital twins can be used to identify high-risk policyholders and proactively offer them risk mitigation strategies, such as installing safety equipment or implementing better security measures.
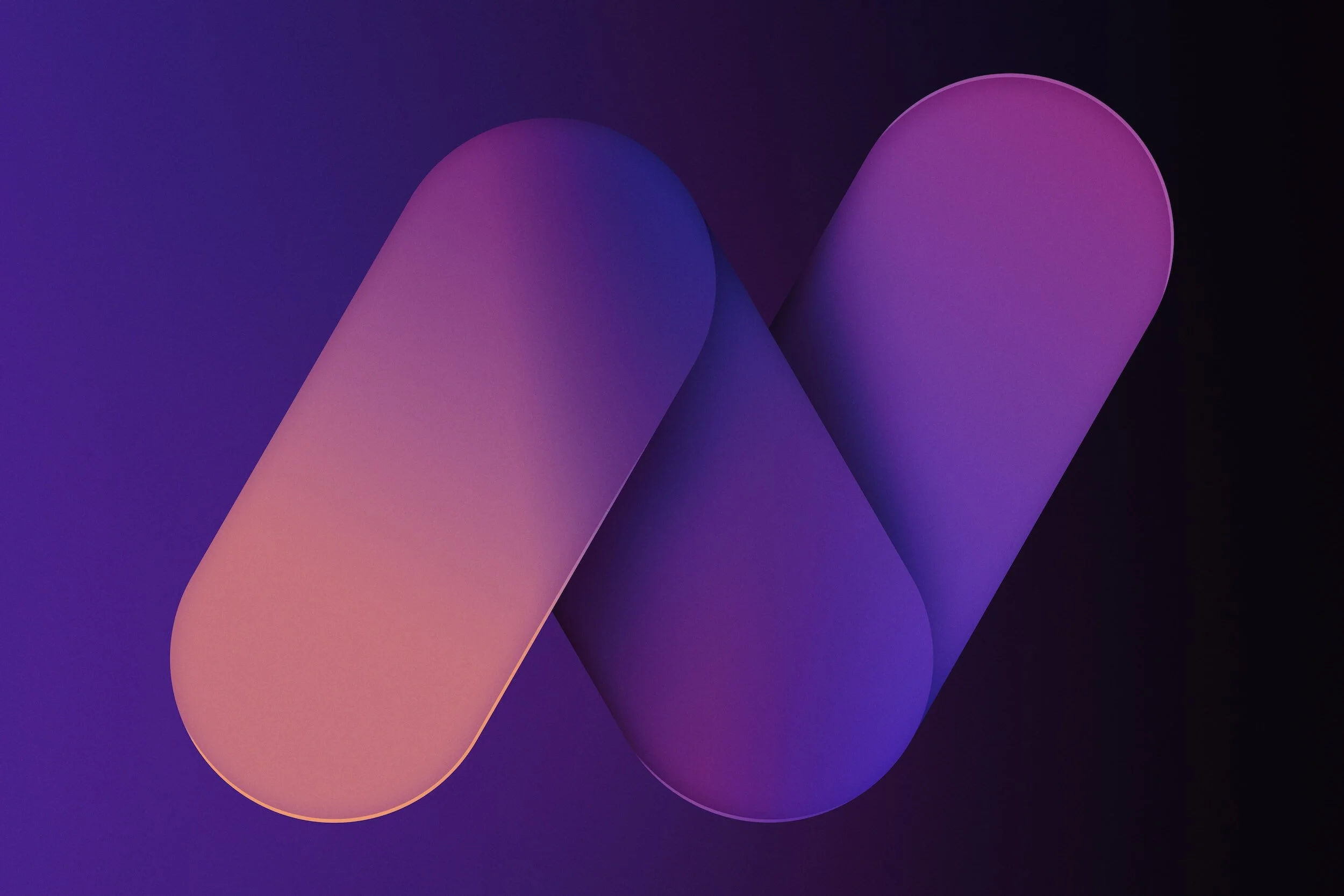
Physics-informed AI digital twins powered by our data apps.
Physics-Informed AI powering the digital twins
Let's start by setting the stage for why the Digital Twin is relevant for the insurance industry. For decades, the power generation industry has collected vast amounts of data, but it was not until later that the terms "digital twin" and "IoT" came into use. However, this data has always been crucial for protecting and controlling the unit. As early as the 2000s, we were using real-time IoT data and analytics to make risk-based decisions for adjusting the engine.
However, over the past two decades, the digital representation of physical assets has undergone significant changes, and the definition of a Digital Twin is a far more precise and advanced representation than others in the industry. Our definition of a Digital Twin is a digital representation of a physical asset, system, or network that precisely simulates reality in a reactive, real-time, bi-directional, synchronous, high-fidelity closed feedback loop process – capturing the real-world physics of a system in a technique called ‘Physics-Informed AI’.
By bringing this definition to life, we can link high-fidelity digital twins together and simulate at both the asset and macroscopic system or plant level. This allows us to better understand failure modes, remaining useful life, and which components across an entire plant become critical junctures of cascading failures.
-
Risk Engineering
Risk engineers can use Physics-Informed Digital Twins to assess the potential risks with industrial assets – risk profiling, surveys, inspections, data collection, sampling, etc. Digital Twins can provide insight into the likelihood of failure and the consequences. Risk engineers can use this information to develop risk mitigation strategies and to recommend changes.
-
Underwriting
Underwriters can use Physics-Informed Digital Twins to more accurately price insurance policies based on the risks associated with specific assets. By modeling the behavior of the assets, underwriters can better understand the potential for losses and adjust policy pricing accordingly.
-
Data Science & Actuaries
Data scientists and actuaries can use Physics-Informed Digital Twins to develop more accurate models of risk and loss. By incorporating data from the digital twins into their models, they can better predict the likelihood and severity of losses associated with industrial assets.
-
CFO & Financial Organization
The CFO and financial organization can benefit from Physics-Informed Digital Twins by having a more accurate understanding of the potential for losses associated with industrial assets. This can help them to better allocate resources and manage financial risk.
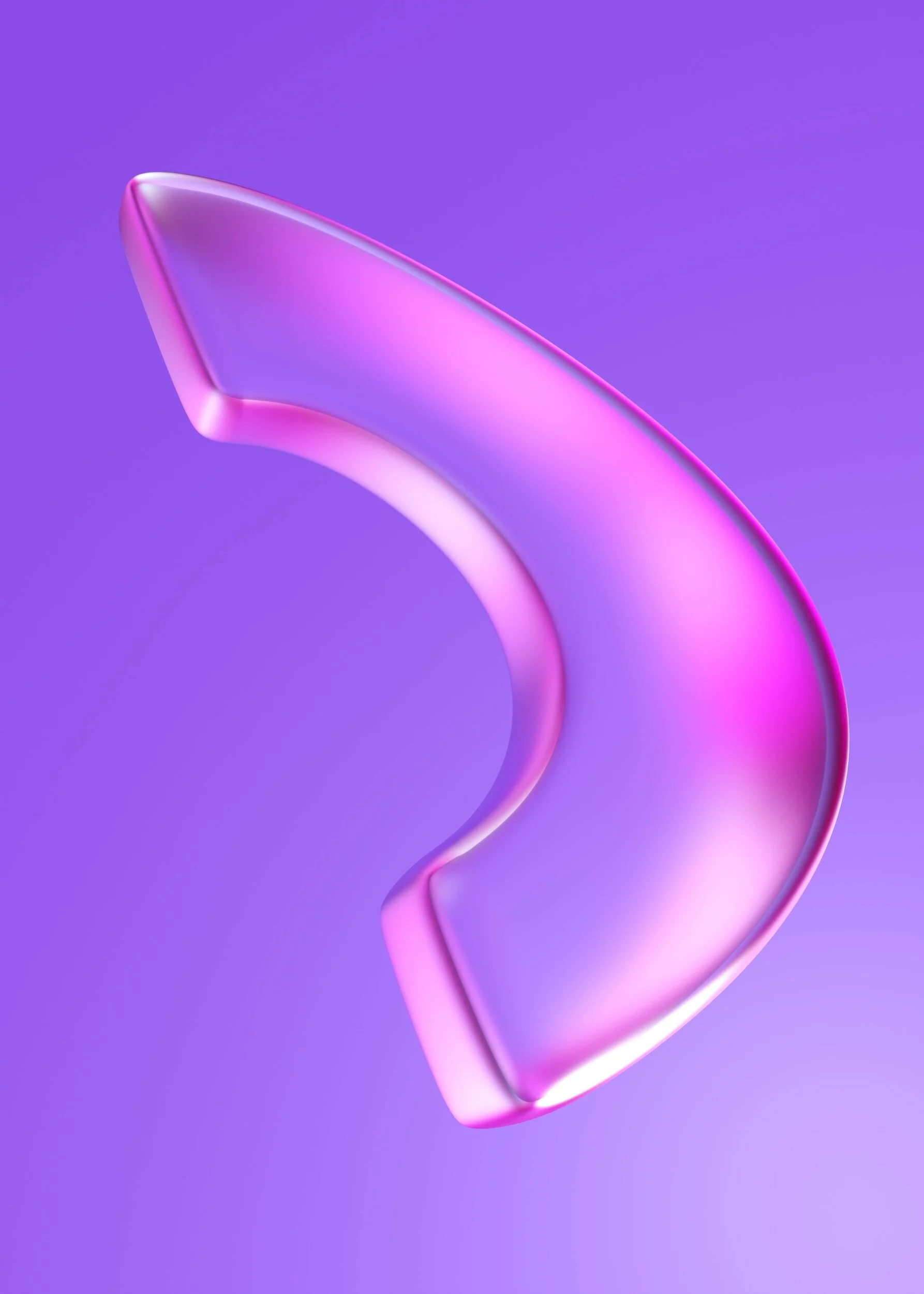
Digital twins powered by an interconnected data fabric to bring the Metaverse to life.
Physics-Informed AI Digital Twins & Data-Driven Digital Twins
In the realm of ‘Physics-Informed AI Digital Twin’, multi-domain physics is the leading edge of the innovation cycle according to Gartner. Although data-driven analytics is a common approach, it lacks the ability to predict failure mathematically and statistically because it is devoid of the physics of the system. In contrast, multi-domain physics models the thermal, fluid, and mechanical conditions of assets under a set of operating and boundary conditions to provide a more accurate performance analysis.
The difference between the two approaches is highlighted below, which shows the conventional data-driven analytics approach and the multi-domain physics approach. The former is characterized by the democratization and commoditization of data science, pre-configuration, and AutoML. It relies on data collection from various sources and analytics engines, which may use pre-defined models of assets and different analytics modes such as anomaly detection and failure prediction. However, it typically lacks multi-domain physics modeling, which is essential for accurate predictive maintenance and forecasting. In contrast, the multi-domain physics approach incorporates physics models of assets to provide a completer and more accurate picture of asset performance.
The multi-domain physics approach starts with the actual physical 1D, 2D, or 3D geometry of the asset as a starting point, taking into consideration the physical parameters, fluid mechanics, and ambient conditions. A reduced order model is then created, which is highly representative of the 3D instance and can run in real-time or in the cloud. This approach is the differentiator and creates more accurate models.
What’s wrong with taking a purely Data-Driven approach?
Current approaches to predicting asset failure through purely data-driven methods fall short in several ways. The question that arises is where the gap lies, and whether it is possible to achieve a predictive state or a forward-looking view of asset failure solely through data. The answer is no, unless Multi-Domain Physics-Informed AI is utilized, rooted in the model itself. This statement holds true for four primary reasons.
Firstly, statistical variation of operating data poses a challenge. If training data only captures normal operations of an asset, how can one predict failure and when it will occur? The statistical variation needed to pick up edge cases or anomalous cases that lead to failure may not be present in the training data set. Things are not failing every day, so predicting failure accurately using a purely data-driven approach is highly unlikely.
Secondly, current models likely only represent singular domain physics, failing to capture the complexities of multi-domain physics interacting with each other across real-world geometry in 1D, 2D, or 3D. In a pure data-driven approach, the physical asset is not modeled in its actual physical instantiation, missing out on thermal, fluid, and mechanical interactions. Moreover, failure does not occur only by modeling current operations and operating data sets. Assets fail via accumulation of thermal stresses and strains, material degradation, and operating hours, which are typically not captured.
Thirdly, much of the analytics available today is linear. Data is processed linearly, and there is no closed loop or ability to affect the control layer. However, a Multi-Domain Physics Informed AI approach goes beyond just modeling assets with higher fidelity, providing the underlying technology stack and IoT stack necessary to control and regulate the Digital Twin of an asset. This approach can lead to self-healing processes, self-regulating assets, self-adjusting, and reactive systems.
Lastly, most of what is done in the industry is at the singular asset level, without any connectivity of the output of one asset algorithm Digital Twin to the next to aggregate performance at the macroscopic level. The lack of a conventional data-driven platform or Industrial IoT Analytics platform to achieve a macroscopic risk profile at the plant level limits the ability to interact and aggregate the performance of multiple assets.
Our data apps.
Our data apps are built on the data fabric for the Metaverse - providing a unified data network, simplified data management, built-in data integration, self-service, centralized data store, and federated governance. Integrated data management for interactive digital twins.
We are building the Industrial Metaverse with Digital Twins-as-a-Service (DTaaS).
Our mission is to convert the industrial landscape into a sea of connected and communicating Digital Twins to de-risk operations for the guardians of our modern infrastructure.